Understanding the Differences: MDM, Data Quality Management, and Data Integration
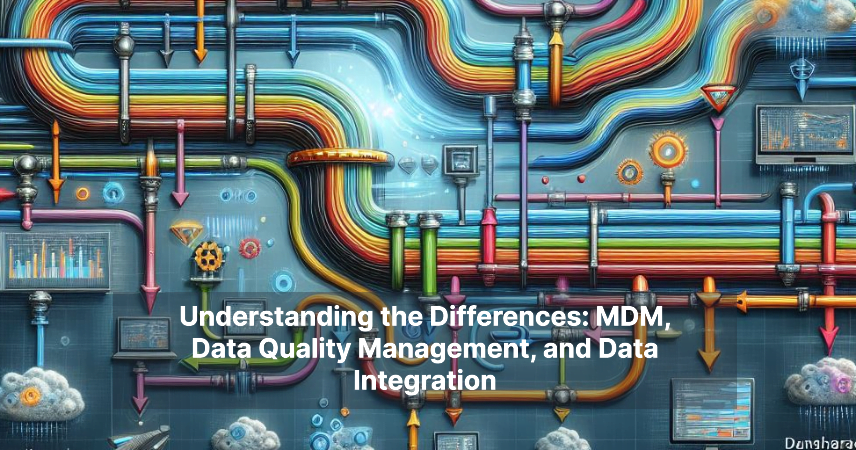
In today’s data-driven world, managing, organizing, and utilizing data efficiently is crucial for businesses of all sizes. Three key components play significant roles in this process: Master Data Management (MDM), Data Quality Management, and Data Integration. While they may seem similar, each serves a distinct purpose in the realm of data management. Let’s delve into the nuances of each.
Master Data Management (MDM)
Master Data Management, or MDM, is a method used to define and manage critical data to provide a single point of reference. Think of it as the backbone of an organization’s data infrastructure. MDM ensures that essential data, such as customer information, product details, or employee records, remains consistent and accurate across all systems and applications.
Key Characteristics of MDM:
- Centralized Data Repository: MDM establishes a central repository where all master data resides, ensuring uniformity and reliability.
- Data Governance: It implements strict governance policies to maintain data integrity, security, and compliance.
- Data Standardization: MDM standardizes data formats, structures, and definitions to facilitate seamless integration and analysis.
- Data Synchronization: It enables the synchronization of data across various systems, eliminating discrepancies and redundancies.
Data Quality Management
Data Quality Management focuses on maintaining the accuracy, completeness, and consistency of data. It addresses issues such as errors, duplications, and inconsistencies that can compromise the reliability and usability of data.
Core Components of Data Quality Management:
- Data Profiling: This involves analyzing data to identify anomalies, inconsistencies, and quality issues.
- Data Cleansing: Data cleansing is the process of detecting and correcting errors or inconsistencies in the data.
- Data Standardization: Similar to MDM, data quality management emphasizes standardizing data formats and structures for improved consistency.
- Continuous Monitoring: This involves continuously monitoring data quality metrics and implementing measures to address any deviations or issues.
Data Integration
Data Integration involves combining data from different sources or formats to provide a unified view. It ensures that data from disparate systems can be accessed, processed, and analyzed cohesively.
Components of Data Integration:
- Data Extraction: This step involves extracting data from various sources such as databases, applications, or files.
- Data Transformation: Data transformation converts extracted data into a common format or structure to enable seamless integration.
- Data Loading: Once transformed, data is loaded into the target system or database for storage and analysis.
- Real-Time Integration: Some advanced data integration solutions offer real-time integration capabilities, allowing organizations to access and analyze data instantaneously.
Understanding the Differences
While MDM, Data Quality Management, and Data Integration share the overarching goal of enhancing data reliability and usability, they differ in their focus and approach:
- Focus: MDM primarily focuses on managing and maintaining master data, ensuring consistency and accuracy across the organization. Data Quality Management, on the other hand, specifically targets the quality aspects of data, addressing issues such as completeness, accuracy, and consistency. Data Integration deals with the technical process of combining data from disparate sources into a unified view.
- Scope: MDM encompasses all critical master data entities within an organization, including customers, products, and employees. Data Quality Management is concerned with ensuring the quality of all data, regardless of its type or source. Data Integration focuses on the technical aspects of integrating data from various sources and formats.
- Approach: MDM adopts a centralized approach, establishing a single point of reference for master data management. Data Quality Management employs a proactive approach to identify and rectify data quality issues continuously. Data Integration utilizes various techniques such as ETL (Extract, Transform, Load) processes to integrate data seamlessly.
Conclusion
While MDM, Data Quality Management, and Data Integration are interrelated components of effective data management, they serve distinct purposes and employ different methodologies. Understanding these differences is essential for organizations to devise comprehensive data management strategies tailored to their specific needs and objectives.